The google definition of novelty is: “the quality of being new, original, or unusual”

Novelty can create a sense of excitement with your audience. The products we’re exposed to daily, from cars, clothing, and electronics, are influenced by new and creative designs to solve a problem. The thought behind this act of design, is you must go to the community you serve, and ask them what they need. But this means that what you create won’t then be novel.
So how do you create novelty that your community will like?
Start to experiment with your community’s viewpoints
and let them define what is novel for them.
Novelty is intricately linked with learning. As humans we are programmed to be more inquisitive about using something new. That is until we get used to it. This is the point where your learned behaviour is accelerated, and that’s when the novelty wears off.
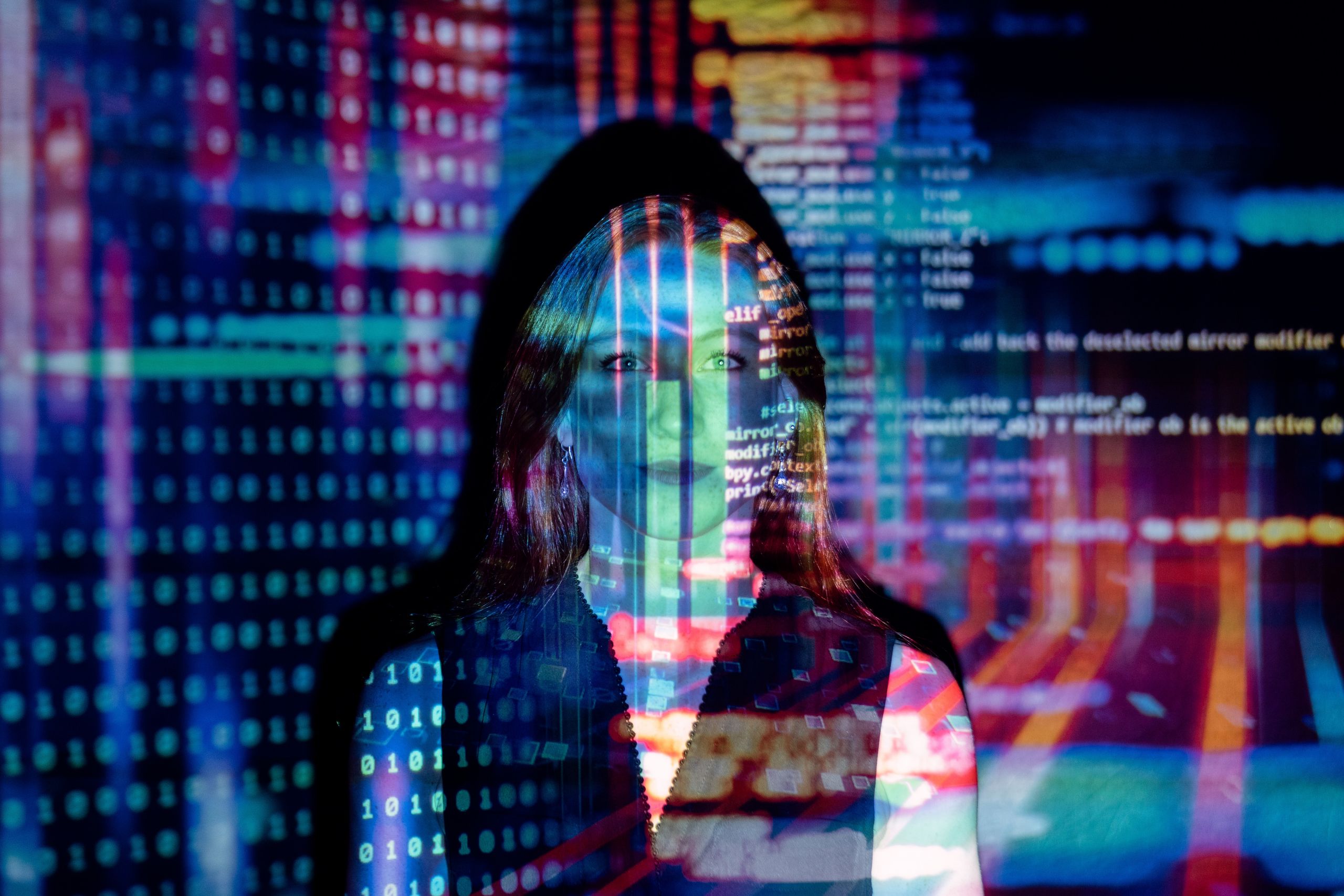
Can novelty be maintained?
Novelty needs to adjust itself to what your community considers as new, and you need to understand that to figure out the right level of novelty.
Recommender systems and engagement digital technologies can help you to learn, and crucially, forget, at your community’s pace.
In a lot of cases, novelty gets accepted because the person was aware of something without really being interested in the topic. People can almost feel scared experiencing something for the first time. A risk sensor kicks in that this experience is too new to the person.
However, if it’s familiar, and still feels novel, it’s likely that the person will engage with that suggestion.
The first time you saw something and decided you didn’t like it; the seed has been planted. The next time you see it, it’s a bit more familiar. The time distance between these first 2 interactions is long. As the time distance gets shorter, there is a construct used to recommend novel things, but it must understand the human condition.
ecosystem.ai build algorithms to figure this out. The customer is presented with options and as they start selecting the things that matter and don’t matter to them, the recommender converges to go down a path that figures out who the person is. If the person is not responding, it tells you something as well. Both reactions sit on the spectrum of analytics to let you understand the person.
But there might be a time that this customer does want something new. This might take a little longer, so the algorithm will still test sporadically, and offer specific recommendations once a month rather than once a day.
How to predict novelty that keeps people interested.
It’s vital to track your community’s behaviour and their personalities in its various forms to build a prediction model that can engage and keep them connected. You will first need an algorithm to engage. But if you don’t know how to go about this, we have a cold start scenario that lets you learn on the fly from the community you serve.
You start off by experimenting with content and observe your customer’s feedback. That feedback provides an input into the next contextual recommender that can recommend specific products, based on what’s learned from the initial context. Letting you set layers of context and automate engagements by just understanding the handful of behaviours in your community.
The future of novel activity is the human being recommended items that are highly specific and personalized to them as individuals. Dynamic experimentation lets you truly see if your community likes your novel suggestions and gives you real results at a minimal cost.