In our last blog post we discussed the importance of creating novelty through experimentation to keep your communities engaged.
Today we’re going to discuss the benefits of low code technology to help data scientists get these experiments and predictions into production faster.
Why choose low-Code technology?
To keep pace with ever-changing human behaviour, you need a technology platform that is reliable, cost effective and can drive a continuous cycle of engagement and experimentation.
There are so many different technologies and algorithms involved in getting prediction models into production, and deployment complexity is continuously increasing. We see a lot of projects fail before getting to production. And for those that make it to that point, the cost is often so high it’s a major showstopper. A low-code environment allows data scientists to take prediction cases and hypotheses to market from beginning to end.
Focus on data science, not technology stack
As a data scientist you need to figure out how to serve your community to drive revenue or stop customers leaving, or whatever scenario your business needs to solve. Using a low-code environment allows you to concentrate solely on data science, rather than on the mechanics and operational infrastructure behind the technologies you use.
Our low code platform gives you this freedom, while also letting you build more human centric engagements or interactions.
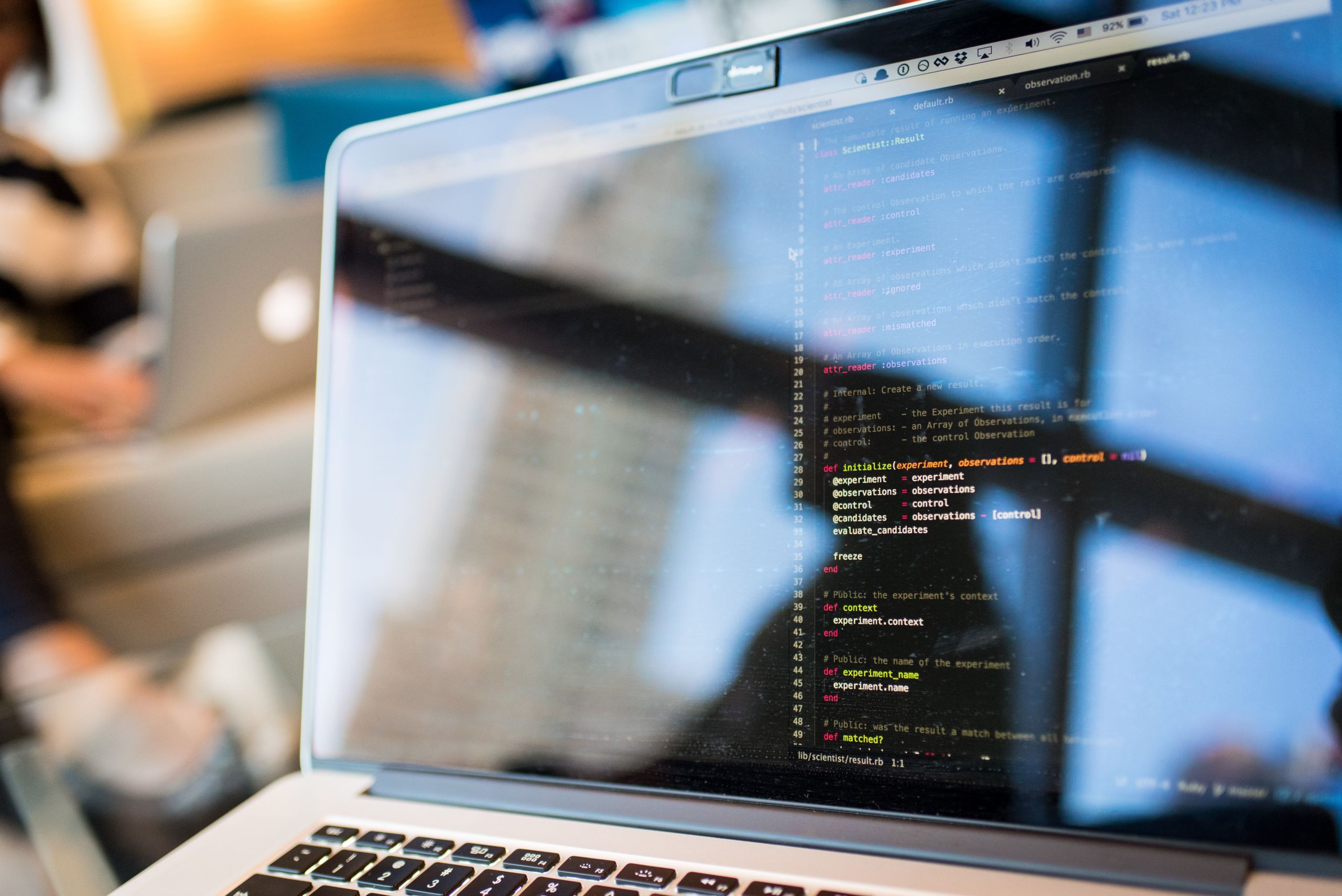
Dynamic recommenders can be built and configured in the workbench within a few hours. This means getting models into production on the same day. Our real-time technology starts to identify patterns and see how people are responding to the recommendations the second they happen, so you can adjust engagements accordingly, in milliseconds.
This lets you create a multitude of contextual models. And each one of those can have its own training criteria and its own recollection. You can even set up models that compete, with some that are trained on historical data, and some that are not. The result is that you can track a human’s engagement over time. You no longer just have a singular dimension; you now have a multi-dimensional view of their engagements with you.
Get your models into production faster
Our low-code platform has 3 tracks that house all the components you need in an integrated architecture:
- The first is a base track that deals with historical data or new data. So you can begin predicting in a cold start scenario if you want. That means you can continuously ingest data throughout the day and night, and your ingestion pipeline can then regenerate or update your option stores.
- The second track is an online engine to update your option stores through ongoing engagement.
- And the third track, is the parameters that you can tweak dynamically while you’re in production, where you can handle your historical data and the time window you need.
The operational environment’s scoring engines and the total implementation sits permanently in production. Meaning you don’t have deployment pipelines that slow you down.
The business interface is where you call the Client Pulse Responder through an API.
The backend parameters that update the scoring engine evolve completely independently from the UI. The back end is your logging dashboard where you track what your performance looks like.
This helps you to move away from the historical process, where you go through an entire batch model training process and then put it into production.
Once your setup is complete, you just need to deploy it. Changes can also be made to your deployments while live in production, without any down time.
With our low-code platform you are permanently in production, and you tweak the parameters to alter the behaviour of engagements while running your live tests and experiments. This lets you be creative with your data science capabilities, without worrying about the infrastructure to support your desired outcomes.
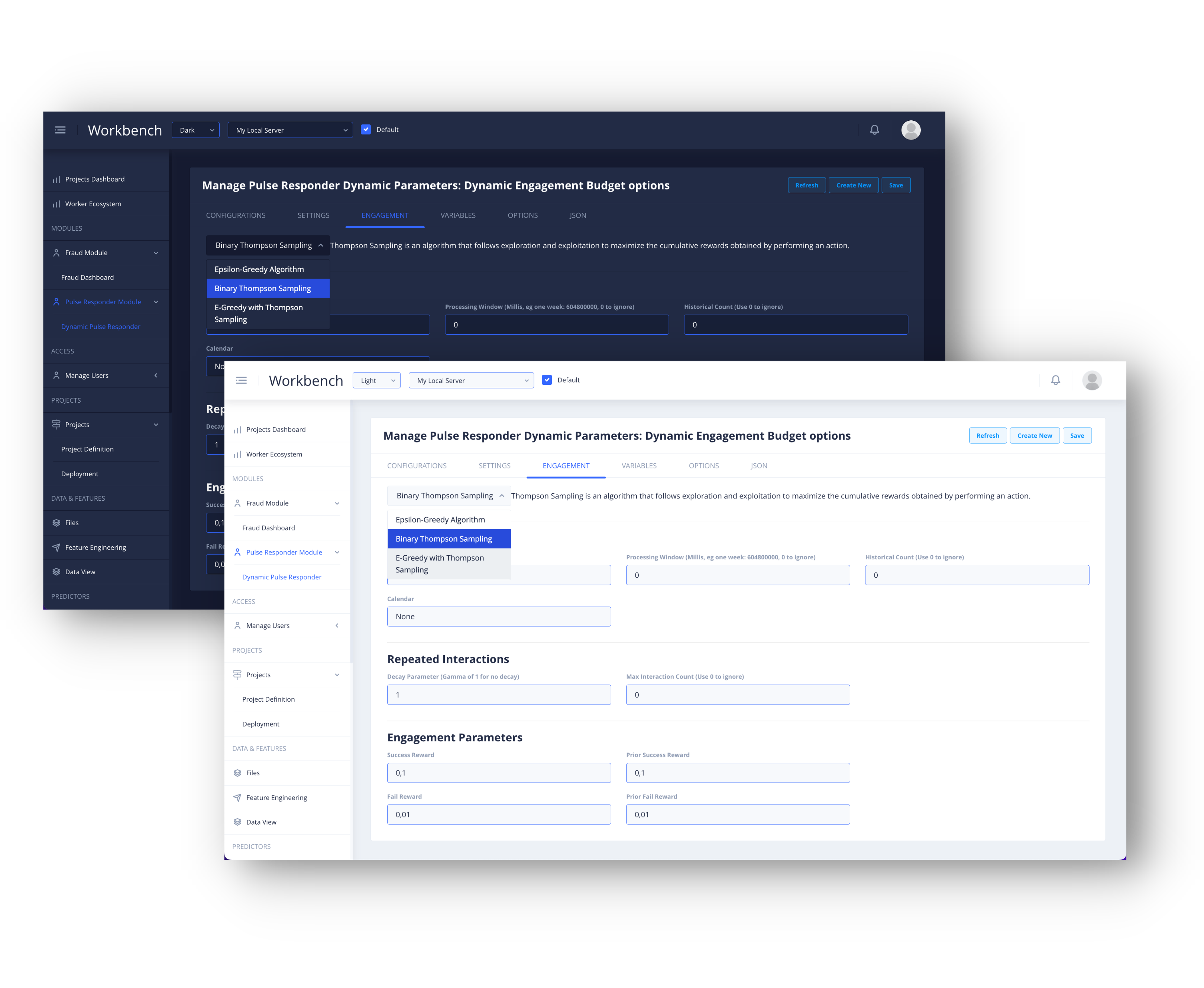