In the tech world, it is becoming increasingly important to account for the human behind the screen, whether these humans are employees, or customers.
Algorithms are still struggling to work accurately for humans. This is due to a lack of innate consideration for the needs of the humans themselves. There is a much higher expectation for machines to get it right, or at least as right as possible.
Alongside this, there is a lot of conversation around data and privacy. Humans want to know where their data is being used. Who it’s being sold to, and most importantly, if they can manage and delete these records themselves.
How do we, as tech creators, ensure the human is always accounted for?
1.
Implement Cultural Knowledge
Humans are cultural beings and as such, are not universal in thier values.
Something important to consider when creating an environment in which personalizing recommendations is the goal, is culture. Oftentimes, it is most beneficial to include ‘culture demographic’ information about a human.
This could be a simple consideration, such as adjusting the words on an engagement message to mirror the colloquial tonality of a given region. It could also be more complex, such as adjusting and testing individualized layouts that best fit a human. It is already a practiced activity in AB testing to offer randomized ‘option A’, ‘option B’ color changes on buttons or text layouts, testing which of the two is the best possible fit for an audience. This can possibly be pushed further, to account for linguistic-cultural color preferences and classifications of a particular group or audience.
Alternatively, attempts could be made to create omni-cultural an recommender environment. Ensuring that interactions or interventions don’t lean in too much to one culture or another. This would require a fair amount of monitoring though, as humans influence the algorithms, and culture influences the human. Understanding audience is the key to fine-tuning an Ai’s cultural sensitivity.
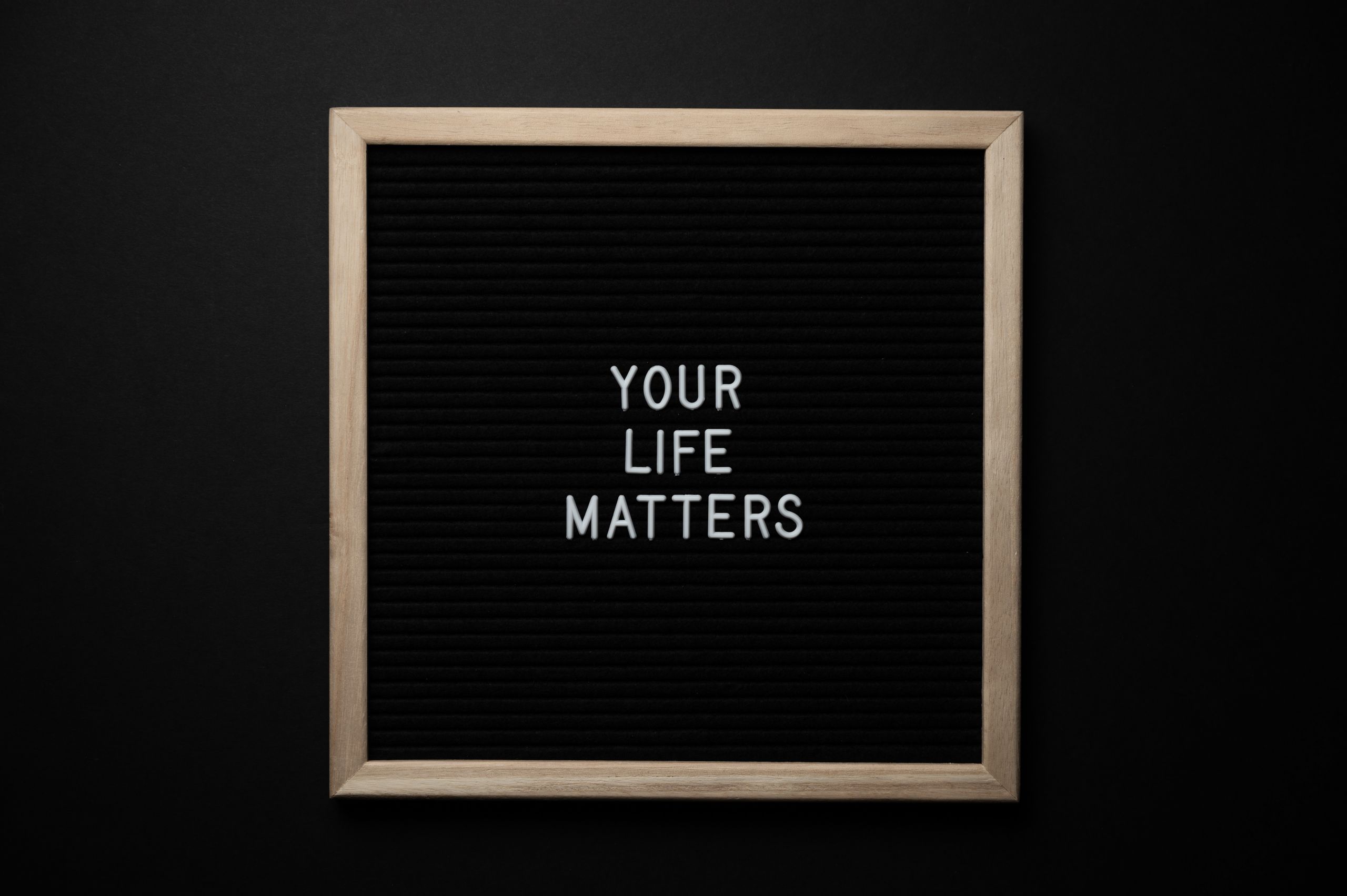
2.
Ensure the Human Feels Valued
This can be done in a variety of ways, but ultimately, humans want to feel that they are important, respected and heard.
Creating on online environment in which the human feels on equal grounding with an engagement is vital. This can be acheived through basic design principles, or language, that make the interaction feel friendly. For example, while the purpose of an engagement defines the tone (such as serious, professional or fun), the choice of words should always be accessible and easily understood.
Additionally, direct interaction with the human, such as when they need help, should always leave them feeling satisfied at the end of the interaction. In order to achieve this, the humans within your support team should feel just as important, respected and heard, as the customers. A versatile chatbot, which is consistently calibrated by humans is also very important. In the end, humans should be interacting with humans, even if indirectly.
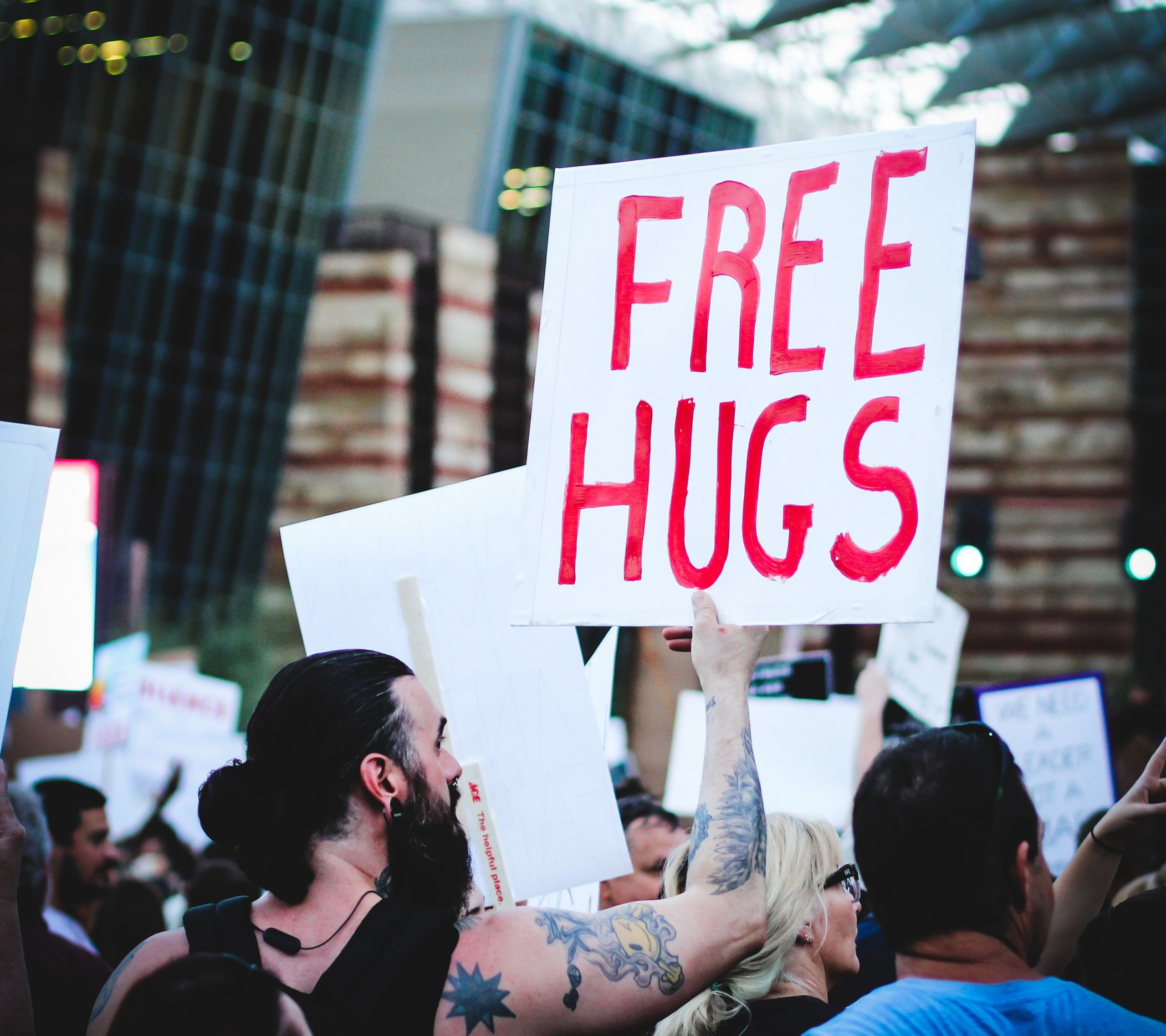
3.
Appeal to Human-like Interests
Humans, at their core, are creatures that put emphasis on symbolism. They will almost always attribute meaning and emotion to abstract things such as art, music, and writing.
This is very important therefore, to keep in mind when appealing to the humans in your system. It is good practice to design engagements, interactions, interventions, environments and more; at a human level. Using visually, emotionally, and mentally enticing imagery, audio, and/or language in order to create a natural appeal to symbolic sentiments.
Essentially, these abstract concepts that drive human emotion also drive human action. Which is the basis for what any tech company thrives on, whether in marketing, predicting, or data analysis. Art and its facets are a fantastic motivator for humans.
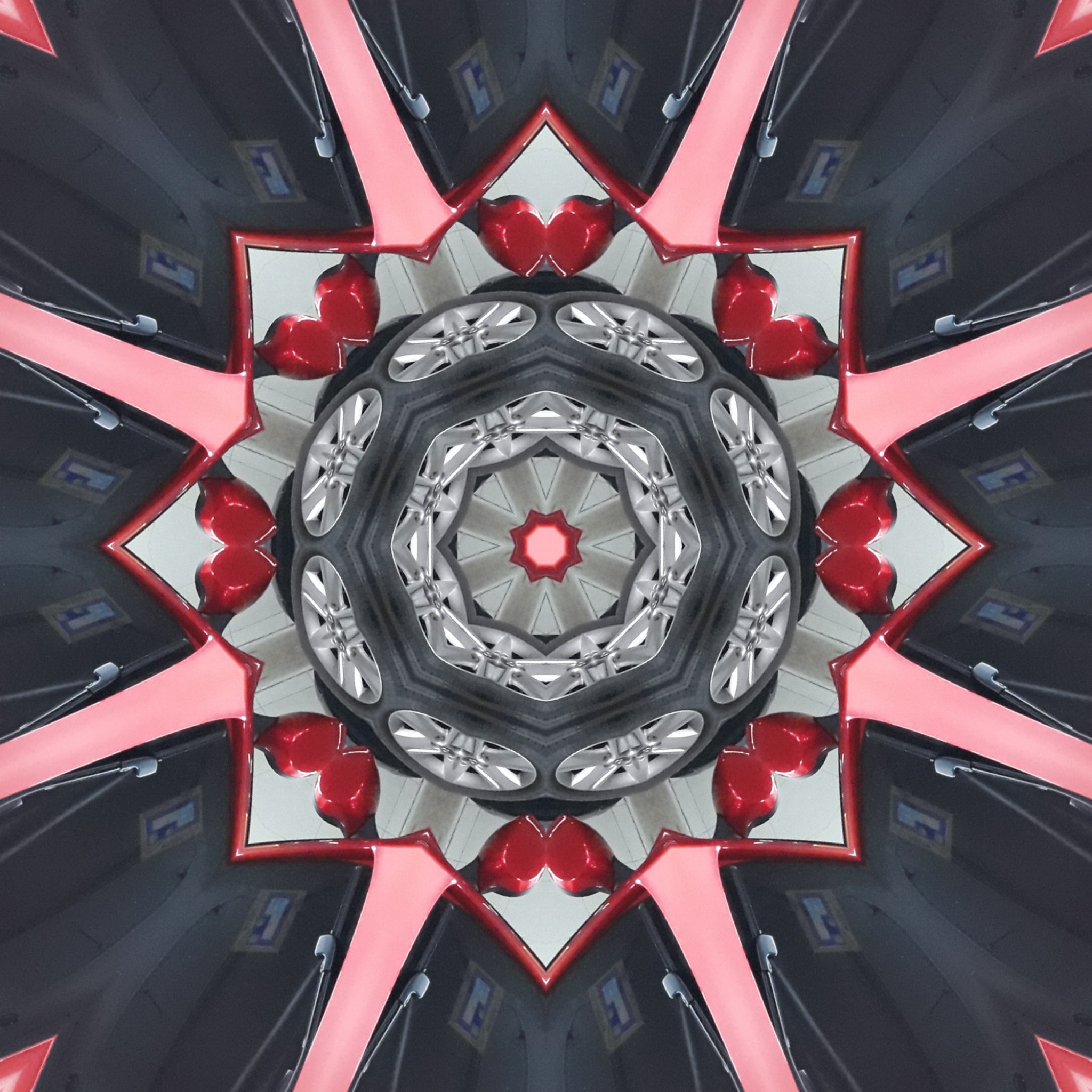
4.
Use Dynamic Experiments to Engage, Without Needing Extensive Personal Data
Within the current global climate, many humans are tired of having their data collected for largely unknown reasons. Until recently, the reasons for, and what types, of data being collected was vague or unexplainable. This is still largely the case.
With Dynamic Experimentation, you do not need access to a humans data to effectively interact with them. Whether your company does not have the data, cannot access it, or what you do have is unusable; you can still engage. This goes miles beyond the capabilities of AB testing, while still maintaining the benefits of the testing process when considering the human.
With this approach, your algorithms learn from and with the humans in your system. Enhancing the effectiveness of your employees by providing them with all the tools they need to amplify the customer experience.
The very nature of Dynamic Experimentation means that you need not worry about humans revoking access to their data, because you don’t always need that personal data. If the cultural considerations, anonymised interactions, and various open tests are in place, every algorithms will adapt and engage without the need for historic data.
The ecosystem.Ai Way
In ecosystem.Ai you can build your own human-centric interactions. Ensuring the cultural attributes of the humans in your system are accounted for by adding culturally relevant features to build your models with.
Encourage a sense of value in employees and customers by tailoring messages and configuring algorithmic attributes in the ecosystem.Ai Workbench. Appeal to human interests and dynamically experiment with various applications to truly account for the human in your system.