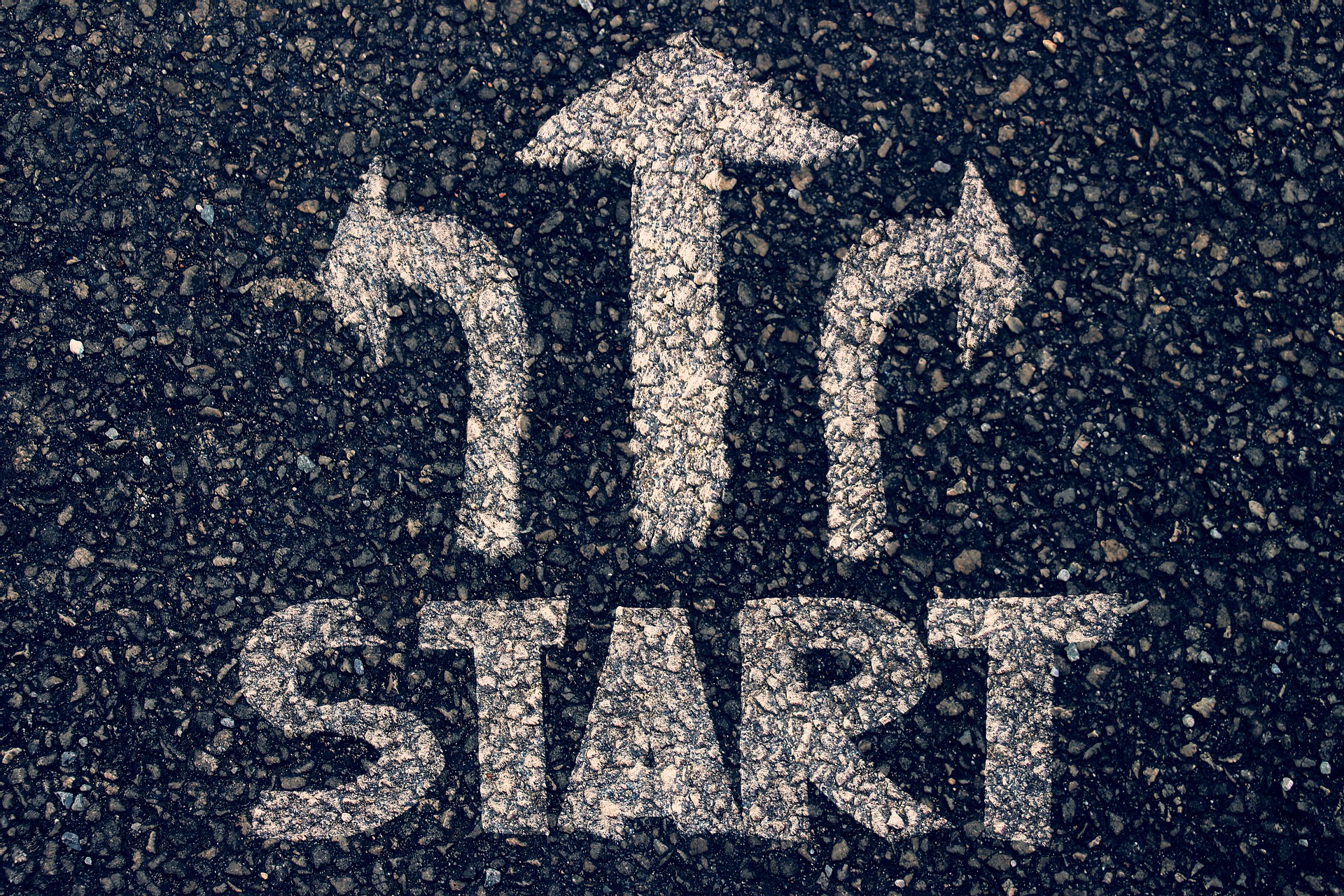
Companies use online experiments to predict the success of products or upgrades before launching to market.
A typical experiment like an A/B test compares 2 versions of something. For example, website design, message text, or headline options are randomly assigned to users to figure out which performs better. Once the best option is determined, results can help companies spot opportunities, mitigate risks, and assess performance in the marketplace.
This can be beneficial when companies have prior product or market knowledge—for instance, an existing product launch into a new market. In this case, understanding the uptake of previous product launches can give you a reasonable indication of the new product’s success.
But consider the scenario where you have no prior knowledge, such as a new product launch into a new market. The options you test will primarily be based on guesswork and gut instinct. Your ability to accurately predict success is greatly reduced so experimentation is essential.
Guess the best. Then experiment with all the rest.
With a traditional data science process, models are built using historical data. At the outset, there may be myriad options identified. But in the case of an A/B test, only 2 options are tested. The outcome of this is to choose one final option for everyone, thereby limiting personalisation with a ‘one-size-fits-all’ approach. By narrowing down your options you never really know if you’ve made the right decision until it’s too late. And whether the other options might have performed better.
Empirical evidence proves this. Even experts get it wrong and are poor at predicting customer behaviour.
Every business has a core product or service they can get historical data on. Customers buy data from telecom companies. They transact using products offered by their banks. But the ability to experiment with all the other things your customer wants over time provides endless opportunities to deliver exciting offers.
This is at the heart of ecosystem.Ai’s dynamic experimentation approach. Recommenders can be set up using a cold start, with no prior data. Real-time scoring engines start testing how people behave based on multiple context variables configured in your dataset. You can see new trends and segments develop and learn dynamically as results unfold in your production environment. You can test all your options at the same time, rather than limit your tests to upfront decisions based on the best prior situation to predict the future.
Let customers real-time interactions shape your business decisions
Our experimentation testing approach is based on presenting virtual widgets (Vidgets) to customers.
So what are vidgets?
They can be anything you want to recommend or rank. Such as products, customer engagement messages, design constructs, special offers, etc.
Rather than selecting just one solution and missing the opportunity to exploit the rest, dynamic experimentation allows you to run multiple tests at the same time. You can test what resonates, what customers like and what they don’t like. And decide to go down a path customers respond to.
Vidgets could be the variations in message text you show to sell a product. Or different design frameworks to show customers when they log onto your app, from heading or text size. Or the order of menu items on your app. The options are endless.
Because you have evidence of real customer uptake and reaction to a range of different options, you are better equipped to come up with new ideas and recommendations. And because you’re experimenting in a production environment, you don’t need a sprint every month to predict what to do next. You test and learn while you engage as your recommender is permanently accessible and continuously configurable.
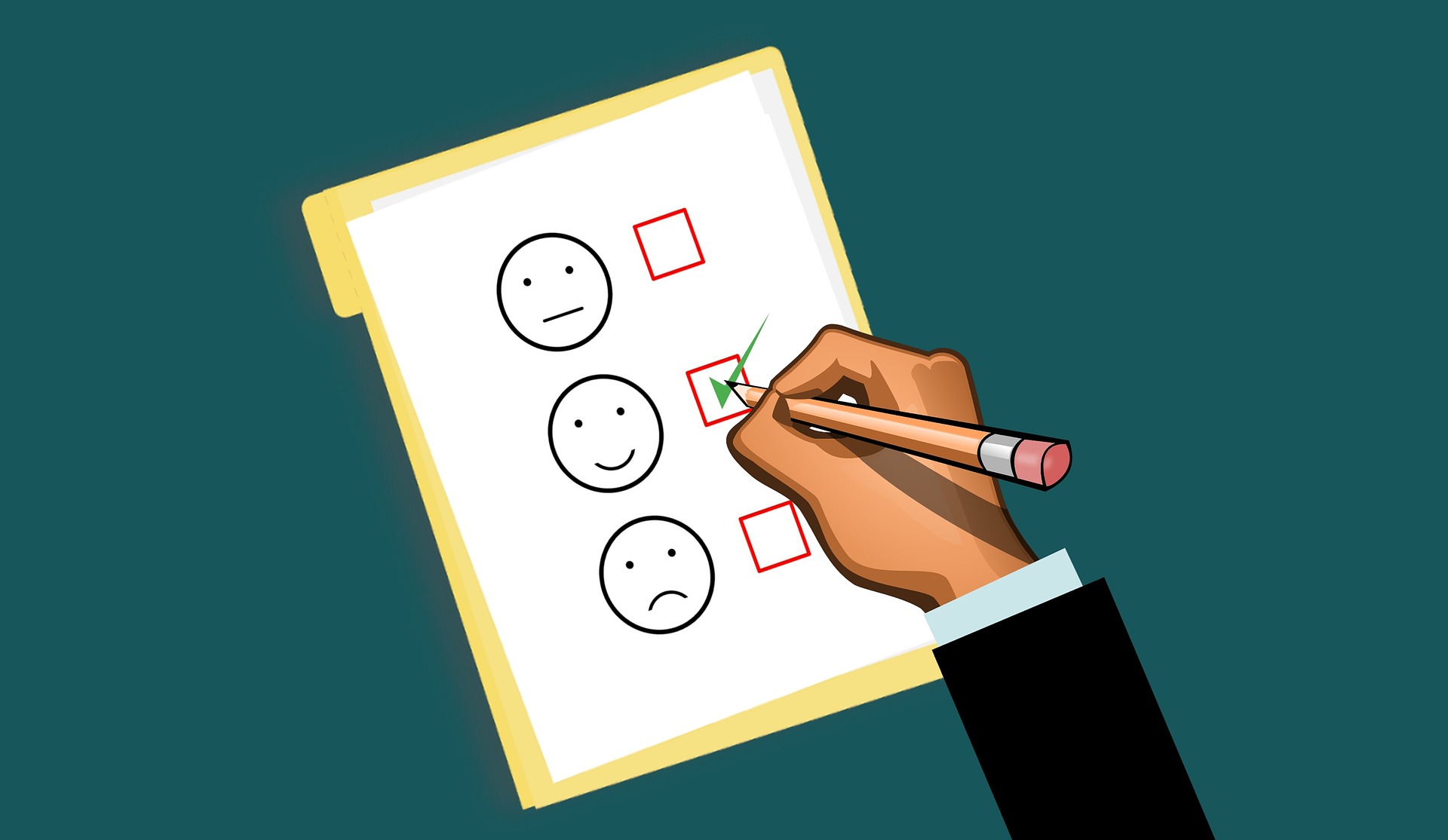
How much can history tell you about your customers’ future behaviour?
Regardless of your launch, whether you have no knowledge yet, or you have prior history of a product or market. You can decide to use that historical data as input or use a cold start with no data. The ability to test all potential options and learn dynamically as the outcomes unfold radically reduces the risk of launching something based on prior history or gut instinct.
This knowledge gives you a much deeper understanding of your customer base and lets you stand out from the competition. Consider how much more insightful and actionable your results would be if you were to run all possible solutions at the same time.