In terms of digital transformation and business innovation, the importance of online testing cannot be stressed enough. Dynamic experimentation can uncover endless opportunities. From acquiring new knowledge, building personalized engagements or offers, to eliminating speculation before product launches or campaigns. The willingness to continuously learn and evolve through time helps companies remain relevant and highly competitive.
“Controlled experiments can transform decision making into a scientific, evidence-driven process—rather than an intuitive reaction. Without them, many breakthroughs might never happen, and many bad ideas would be implemented, only to fail, wasting resources.”
Experiments bring tangible evidence early in the process when it is still possible to change the direction without big costs. Therefore, the earlier we start experimenting and collecting information, the quicker we can reduce the level of uncertainty.
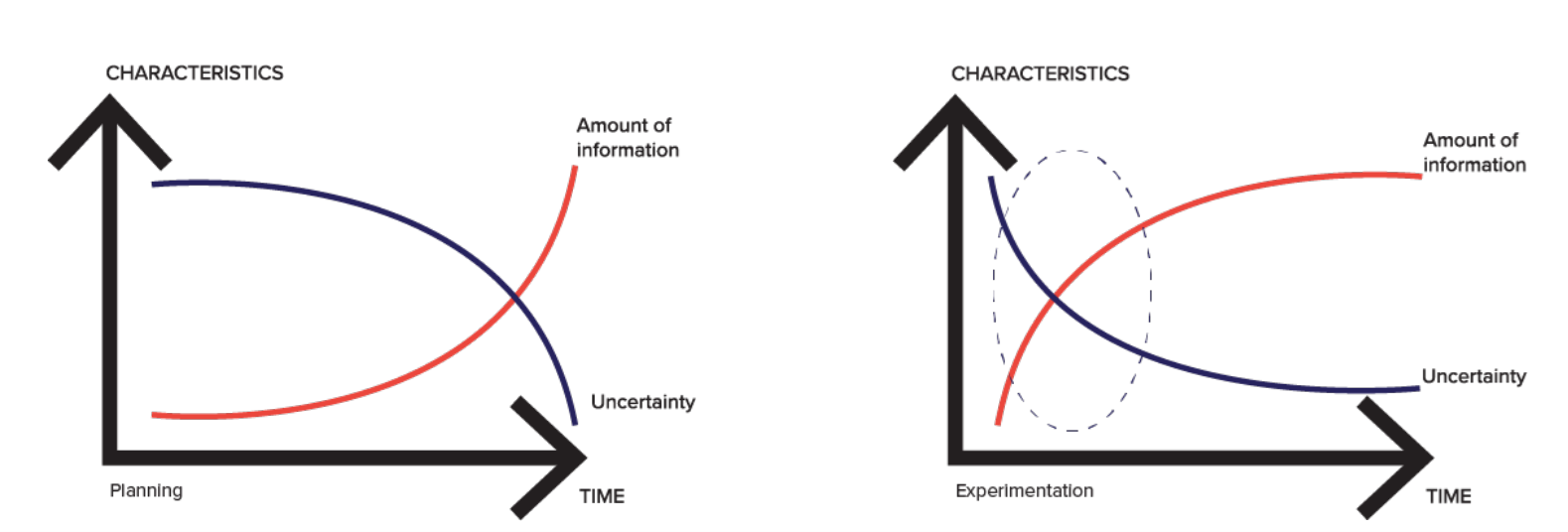
But so many companies have yet to adopt experimentation or have implemented arbitrary testing approaches that end up with skewed results and a poor return on investment.
Let’s consider some of the reasons for that and uncover how dynamic experimentation can overcome these issues.
Can history be a true representation of the future?
Classical machine learning processes tend to take a waterfall approach. An end-to-end process might start with a data set from a point in time, which is put through feature engineering with additional features added, before the model is trained. Then some code is wrapped around it, and it’s deployed to production.
This may work ok where you have collected data for this scenario before, say an existing product launch into an existing market. But because the approach assumes static behaviour after that point in time, it can’t account for customers changing behaviour, their most recent transactions or rituals and events.
It’s also can’t help you predict a scenario where you don’t have any data points already based on historical behavior, such as launching a new product into a new market. Experimentation is crucial in this case, to determine how to engage with your audience, and to predict how they will respond.
To gain new knowledge we must experiment and evolve
A successful dynamic experimentation approach can only be achieved through real-time analysis of interactions and engagements while models are running in production. This allows for new knowledge acquisition. And for far more accurate and diverse engagements to know what that human on the other end is going to do when you present them with different options.
Because you’re already interacting with people while running in production, you can quickly check that you’re making the right decisions. And that your model can converge based on the criteria that you set, without having to go through this lengthy data science cycle that normally comes with preparing these kinds of recommenders.
Building an experimentation pipeline
When considering how to setup a dynamic experiment we propose 5 levels of testing to ensure you are deploying optimal recommenders to production. These are:
-
Cold Test
More of a classical measure, than dynamic, this is where you would look at engagement recall and accuracy.
-
Warm Test
This is where you can configure different parameters, input your data, or link to a feature store and see if the prediction results make sense to you. If not, this phase gives you the opportunity to delve deeper and figure out what’s going on, or if there’s a mistake somewhere that is impacting results.
-
Simulation and Dynamic Engagement
Simulations are immensely useful, they’re the first step where you can validate that you’re doing the right thing. They’re not only useful for you to interpret results, they’re also essential to figure if the performance, security, and downstream execution is all in place.
-
New to Production
This phase is where you launch your experiment, whether that’s a campaign, new recommender, intervention, or a product offer into production. The real time capability lets you track so many different criteria using dashboards and other tracking tools.
-
Continuous Learning
Now that the simulation and move to production phases are complete, this is where you continuously track the recommender you’re deploying or alter the behavior of the interaction you’re testing. This approach happens in production, without ever having to go back into your pipeline.
Once you have the right tooling in place, dynamic experimentation gives you the capability to keep learning more about the people you engage with. Armed with this new knowledge you can build more personalized and relevant interactions without having to go through a more traditional heavy productionization process.